Generative AI Models Face Challenges with Tokenization and Language Biases
Background
In recent years, generative AI models have revolutionized various industries by enhancing natural language processing and text generation capabilities. However, these advancements also highlight significant challenges and biases inherent in the tokenization process.
As global demand for multilingual AI solutions grows, addressing these inefficiencies becomes crucial to ensuring fair and equitable access to AI technology across diverse linguistic communities.
News Summary
Generative AI models, like GPT-4, process text through tokenization, breaking it down into smaller units like words, syllables, or characters. This method allows models to handle more information but introduces biases, inconsistencies, and inefficiencies, particularly with non-English languages and numerical data.
Tokenization can lead to higher costs and poorer performance for users of certain languages. Alternative models like MambaByte, which process raw text without tokenization, show promise but are still in early research stages and face computational challenges. Future advancements in model architecture may be necessary to overcome tokenization limitations.
Personal Insights
The article highlights several critical aspects of generative AI models, particularly focusing on the tokenization process and its associated challenges.
Technical Perspective
From a technical standpoint, tokenization is both a strength and a limitation of current AI models. It allows models to process and generate text efficiently by breaking it into smaller, manageable units.
However, this same process introduces biases and inefficiencies, particularly with languages that have different structural rules compared to English. The inconsistency in tokenization, such as treating “HELLO” as multiple tokens while “hello” is one, can lead to performance issues. Furthermore, the difficulty in processing numerical data and handling special characters reveals the inherent limitations of token-based systems.
Linguistic Perspective
Linguistically, the article underscores a significant bias towards English, as many tokenization methods were initially designed with English in mind. Non-English languages, especially those that do not use spaces between words or have complex writing systems, face substantial inefficiencies.
This not only impacts the accuracy and performance of AI models but also increases the cost for users of these languages due to the higher number of tokens required. This linguistic bias poses a challenge to the equitable development and deployment of AI technologies globally.
Ethical and Social Perspective
Ethically, the article highlights issues of fairness and inclusivity. The current tokenization methods exacerbate language inequities, making it essential to develop AI technologies that are accessible and beneficial to all linguistic communities.
The potential for bias and the higher costs associated with non-English languages raise concerns about the inclusivity of AI advancements. Ensuring that AI development is equitable and does not reinforce existing social divides is crucial.
Research and Innovation Perspective
The discussion on alternative models, such as MambaByte, which bypass tokenization by processing raw text data directly, is promising. These models could potentially address many of the current limitations of token-based systems.
However, they are still in the early stages of research and face significant computational challenges. Continued innovation and research in this area are essential to overcome the limitations of current AI models and develop more efficient, fair, and inclusive technologies.
Conclusion
In conclusion, while generative AI models like GPT-4 have made significant strides in natural language processing and text generation, the inherent challenges of tokenization highlight the need for further research and development.
Addressing these issues from technical, linguistic, economic, ethical, and innovative perspectives is crucial to ensuring that AI technologies are efficient, fair, and inclusive for all users, regardless of their language or socio-economic background.
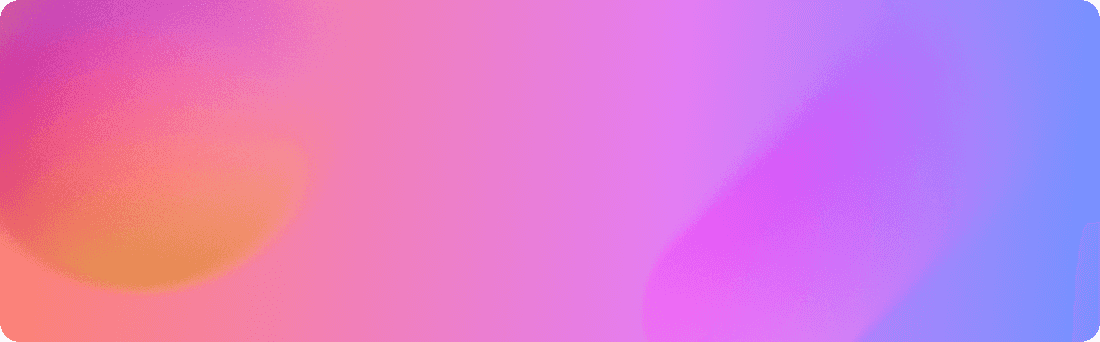
Related Articles
Cloudflare's New Tool Targets Unauthorized AI Data Scraping
Guarding the web: Cloudflare's new tool tackles AI bots scraping data, raising vital questions about ethics, data ownership, and the future of AI.
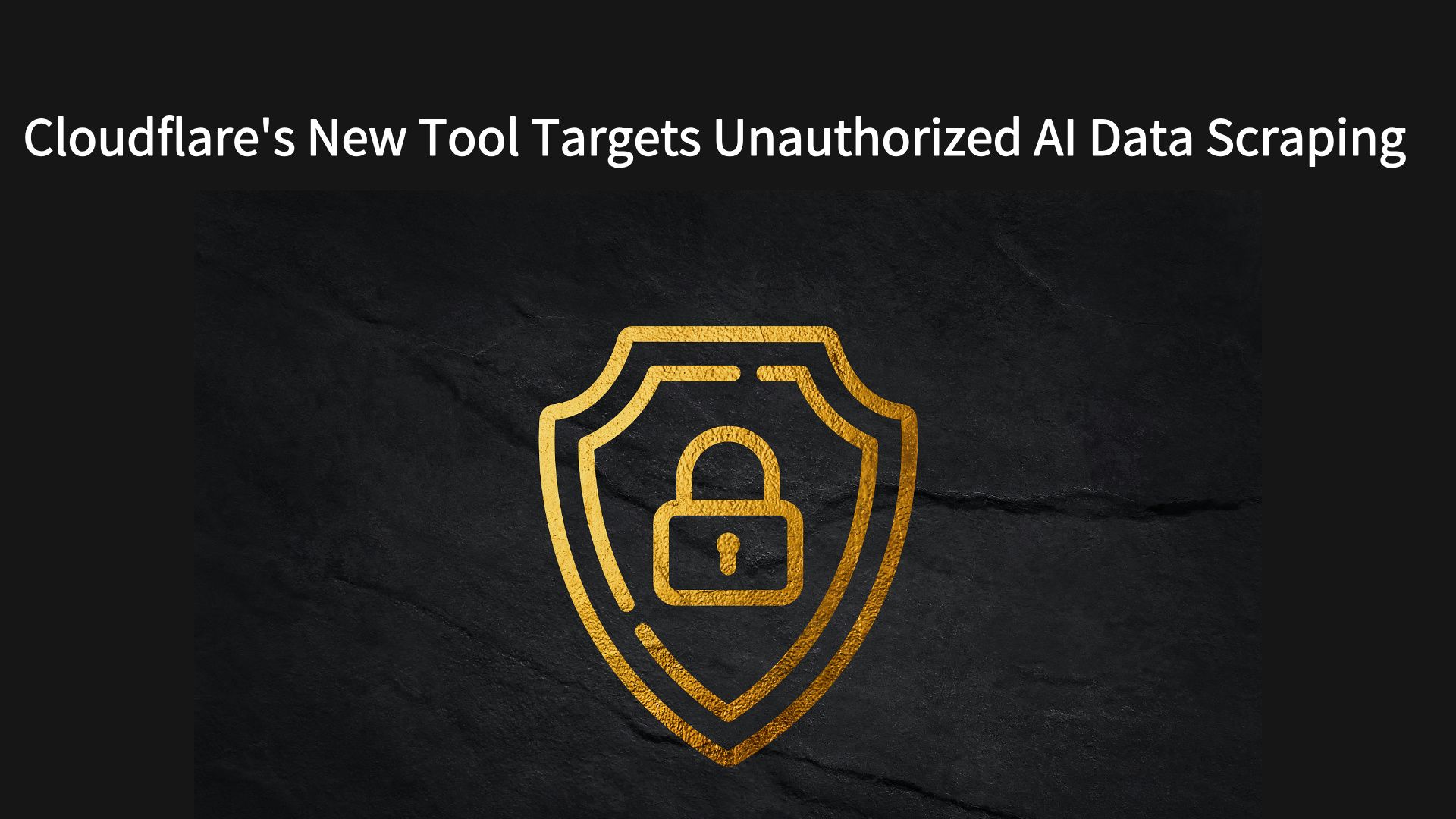
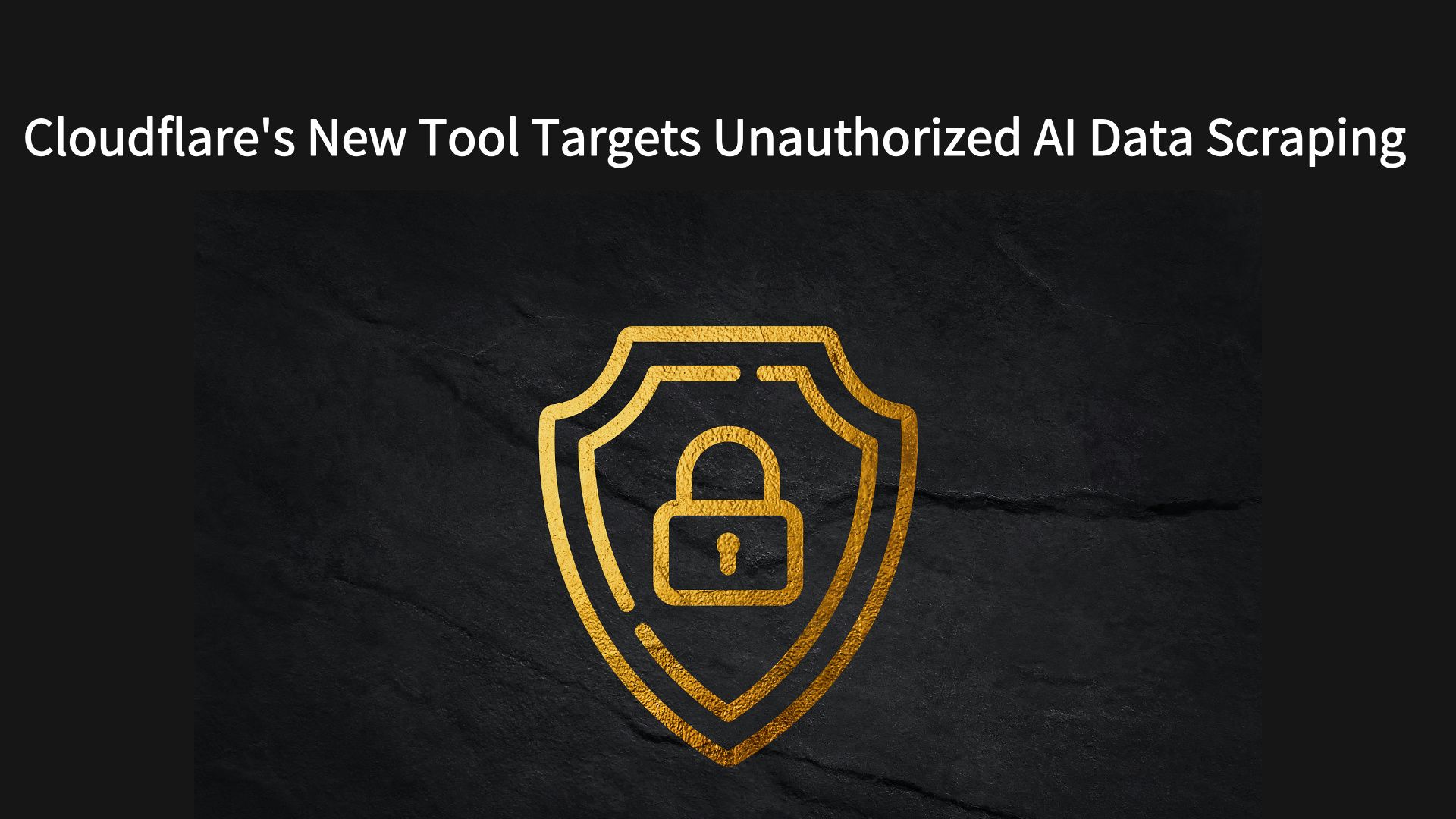
AI Therapists: Bridging Gaps in Mental Health Care Amid Rising Demand
Explore how AI therapists are transforming mental health care by providing scalable, affordable support, and learn about the technological, ethical, and practical considerations shaping this innovative approach.
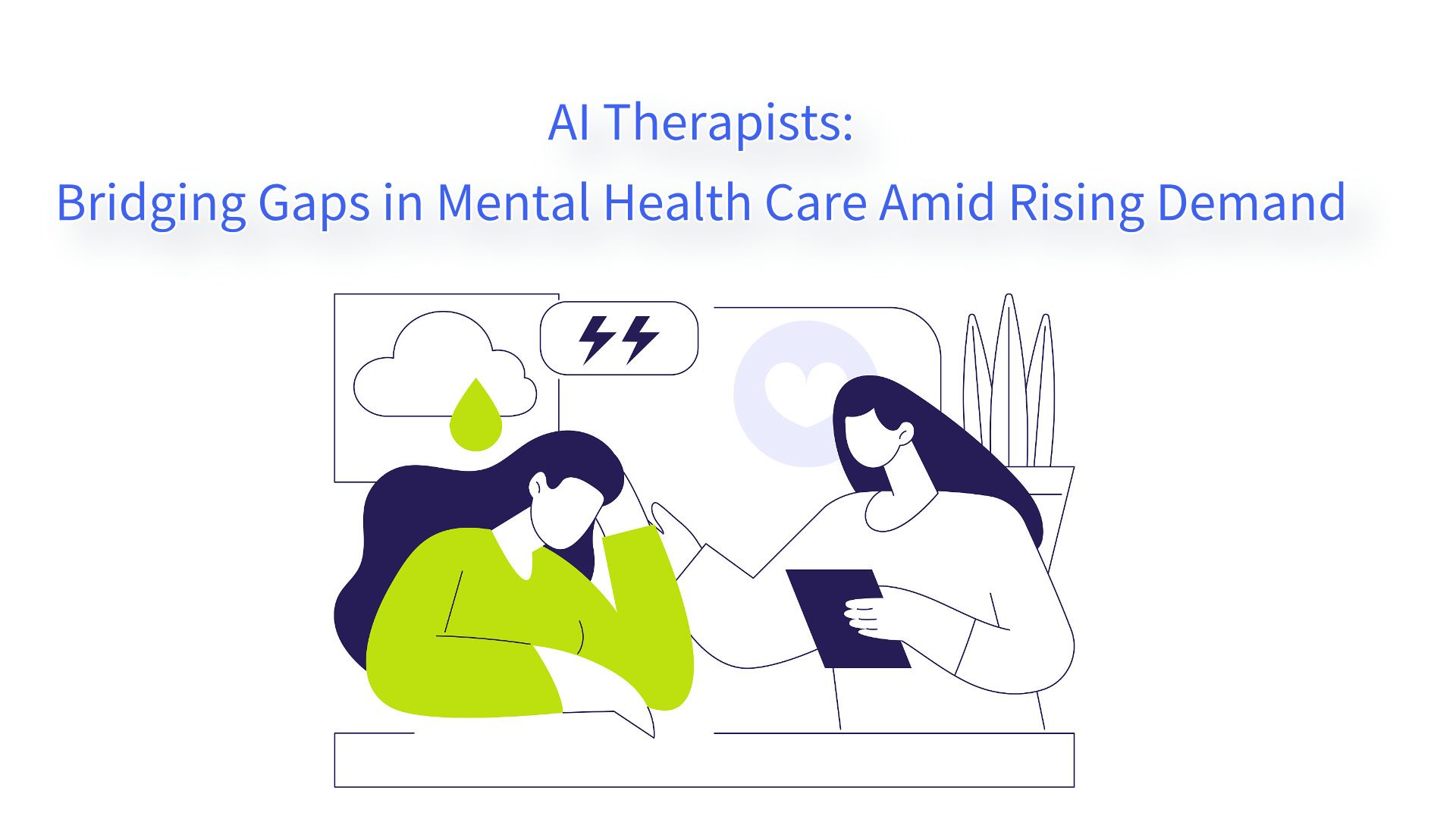
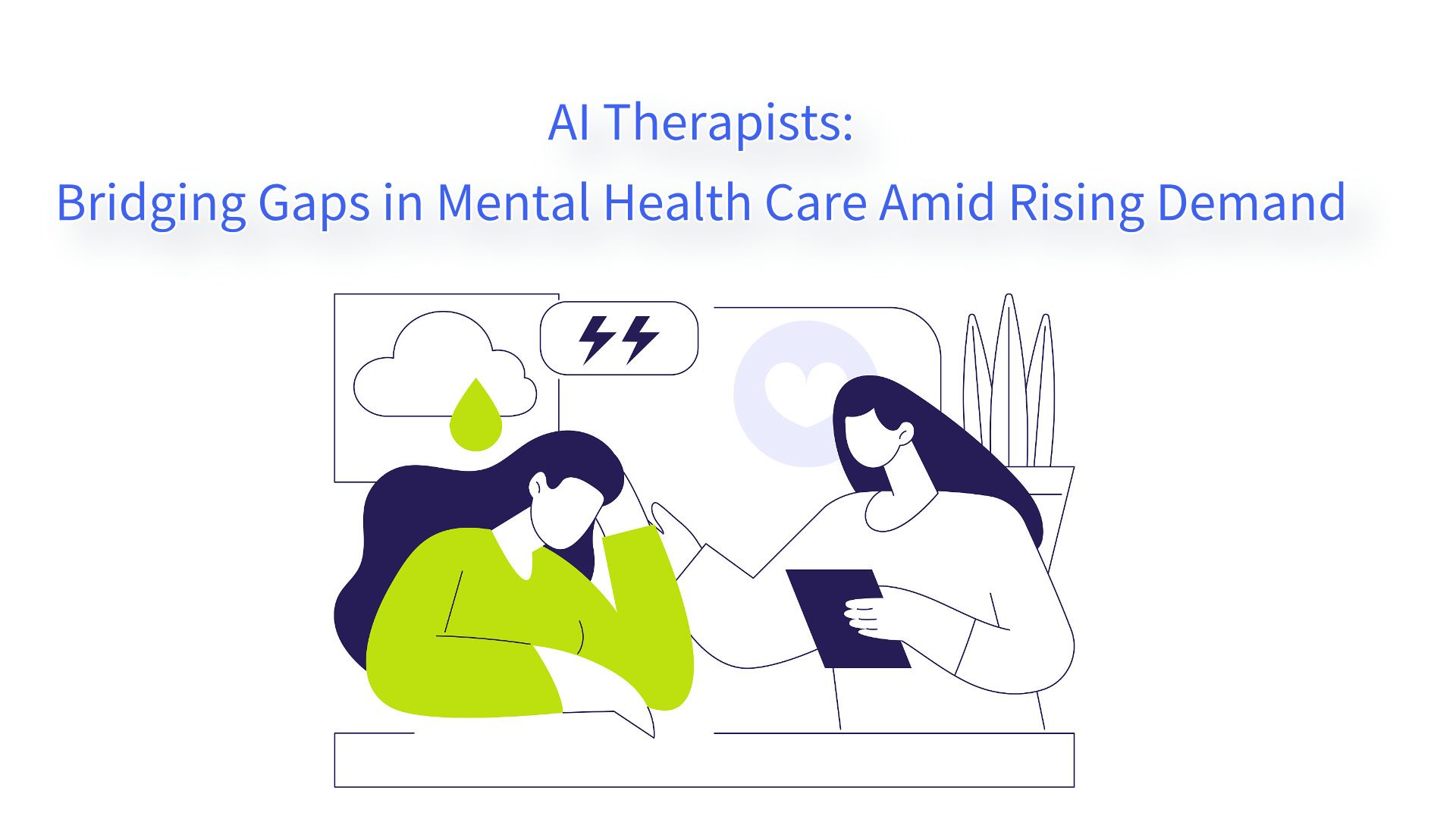
Robotic Pets Combat Loneliness Among Aging Population
Explore how robotic pets are revolutionizing elder care, offering companionship and emotional support to combat loneliness in aging populations worldwide, and discover the exciting technological and ethical implications of this innovative trend.
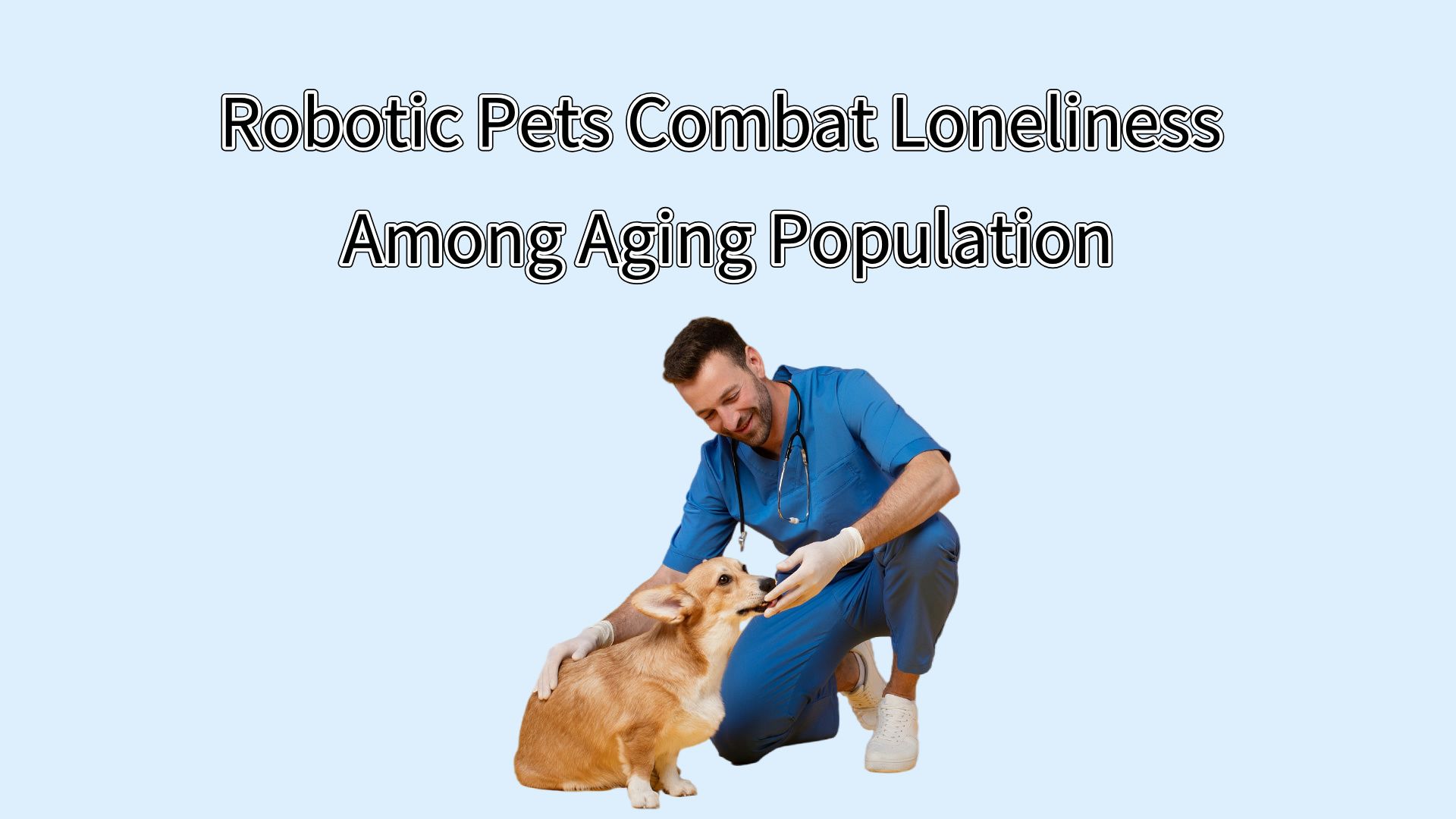
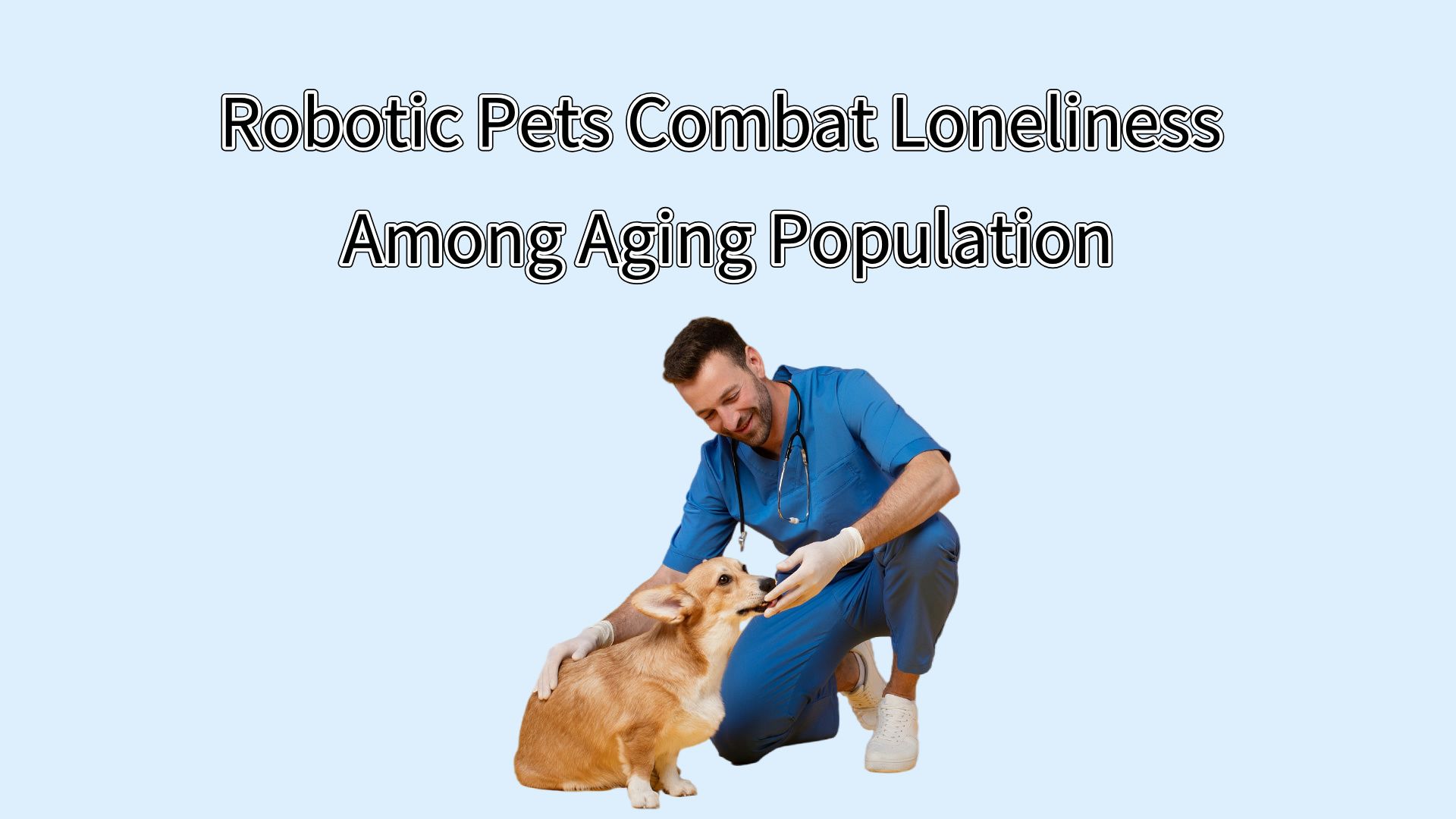